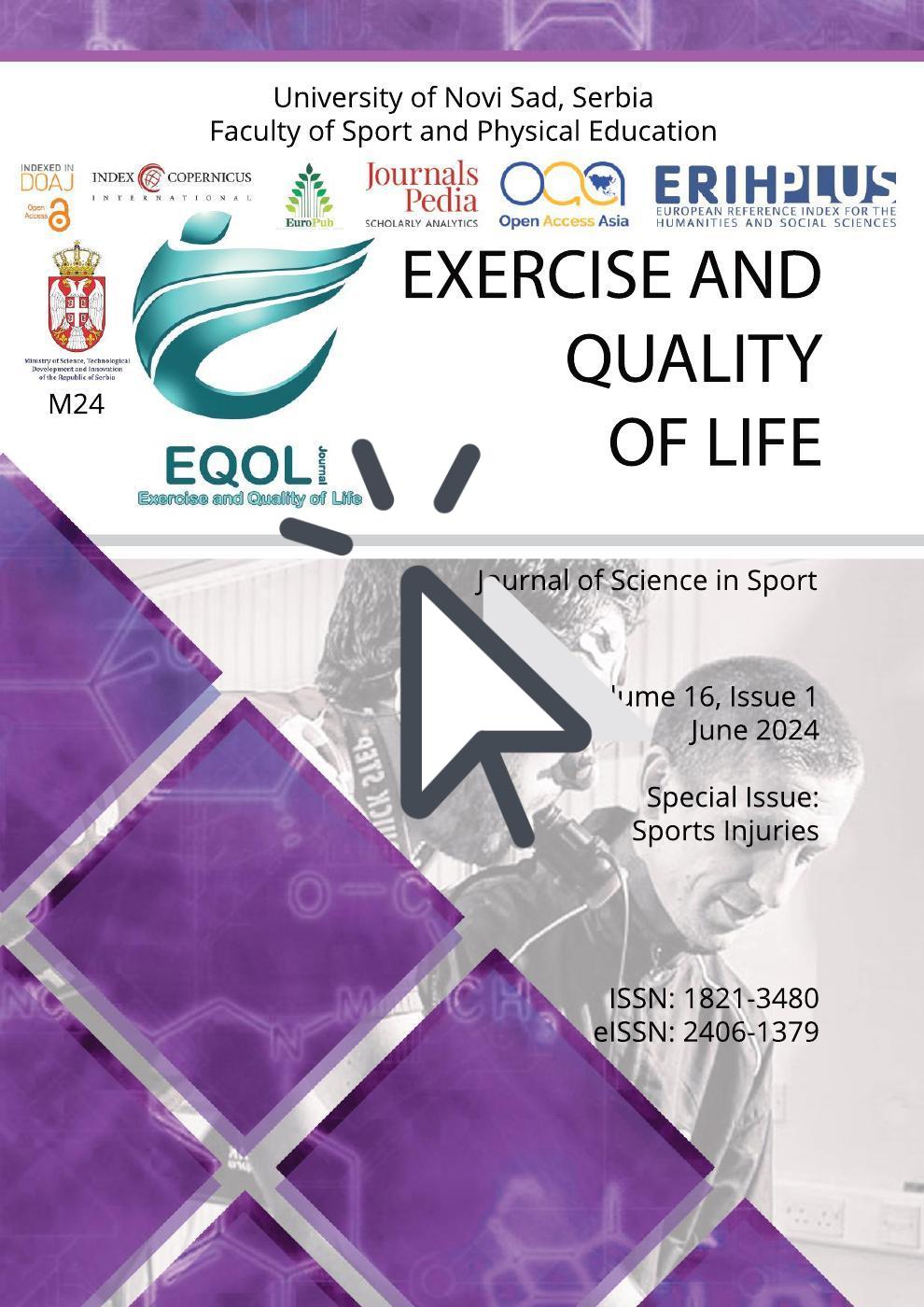
More articles from Volume 3, Issue 1, 2011
NUMBER OF STEPS PER DAY AND PHYSICAL ACTIVITY LEVELS OF ADULTS IN GREECE
AN AUTOMATED IDENTIFICATION OF INDIVIDUALS AT HEALTH RISK BASED ON DEMOGRAPHIC CHARACTERISTICS AND SELF-REPORTED PERCEPTIONS
CANDIDATE GENES IN THE FIELD OF EXERCISE GENOMICS
THE EFFECT OF STRENGTH TRAINING ON TENNIS SERVICE PERFORMANCE OF JUNIOR TENNIS PLAYERS
RELATIONS BETWEEN ANTHROPOMETRIC CHARACTERISTICS AND LATENT DIMENSIONS OF STRENGTH IN PERSONS OF ABOVE-AVERAGE MOTOR ABILITIES
AN AUTOMATED IDENTIFICATION OF INDIVIDUALS AT HEALTH RISK BASED ON DEMOGRAPHIC CHARACTERISTICS AND SELF-REPORTED PERCEPTIONS
Department of Health Studies, Eastern Illinois University, Charleston, United States
Center for Bioinformatics and Computational Biology, University of Maryland, United States
Department of Public Health, The University of Texas at El Paso, El Paso, United States
Published: 01.06.2011.
Vol 3, No 1 (2011), 2011, 3 (1);
pp. 11-21;
Abstract
The risks of developing diabetes, high blood pressure, and cardiovascular disease could be reduced by increasing the number of individuals receiving adequate levels of physical activity (PA). Centers for Diseases Control and Prevention (CDC) has reported that about 30% of Americans do not engage in any PA and about 40% engage in some levels of PA, but still not meeting the recommended levels defined by the American College of Sports Medicine (ACSM). Studies have shown that the greatest declines in PA occur during the transitions from high school to college and beyond. Thus, it is important to identify students at young age that are at health risk due to lack of PA, so that specific steps could be taken toward helping these individuals develop a healthier lifestyle. We used data on 100 college students to develop a preliminary computer program (using a backpropagation multilayer neural network approach) to automatically identify individuals at risk of being not sufficiently physically active. Besides various types of demographic variables, data included information on the association between studentsí self-reported levels of PA and Social Cognitive Theory (SCT) constructs (e.g., self- efficacy, self-regulation, social support, expectations), as predictors of participation in PA. The results of this study indicated that the backpropagation multilayer neural network identified and classified individuals at risk of being not sufficiently physically active into right categories (atrisk individuals or not at-risk individuals) 77% of the time. Collecting additional data points that contain more at-risk individuals will improve the neural network's prediction of at-risk individuals.
Keywords
References
Citation
Copyright
This work is licensed under a Creative Commons Attribution-NonCommercial-ShareAlike 4.0 International License.
Article metrics
The statements, opinions and data contained in the journal are solely those of the individual authors and contributors and not of the publisher and the editor(s). We stay neutral with regard to jurisdictional claims in published maps and institutional affiliations.